The 2025 CVPR EarthVision Data Challenge – Pushing the Boundaries of AI-based Compression in Earth Observation
As part of our commitment to advancing AI-driven geospatial analytics, the Embed2Scale consortium is thrilled to organize the 2025 CVPR EarthVision workshop Data Challenge. This challenge invites researchers and AI practitioners to develop cutting-edge Lossy Neural Compression for Geospatial Analytics techniques, optimizing how Earth Observation (EO) data is processed and utilized.
Co-sponsored by Embed2Scale and the IEEE Geoscience and Remote Sensing Society, this year’s challenge is designed to push the limits of self-supervised learning (SSL) and AI-powered embeddings. Participants will work with SSL4EO-S12 data cubes, creating highly efficient neural encoders to compress EO data while maintaining its analytical utility for downstream applications.
The challenge
More specifically, we ask you to develop an encoder E, a.k.a. pre-training, to compress SSL4EO-S12 (v1.1) data cubes D down to 1024 features X, a.k.a. embeddings, that we evaluate on a hidden set of applications, a.k.a. downstream tasks as in the literature of foundation models.
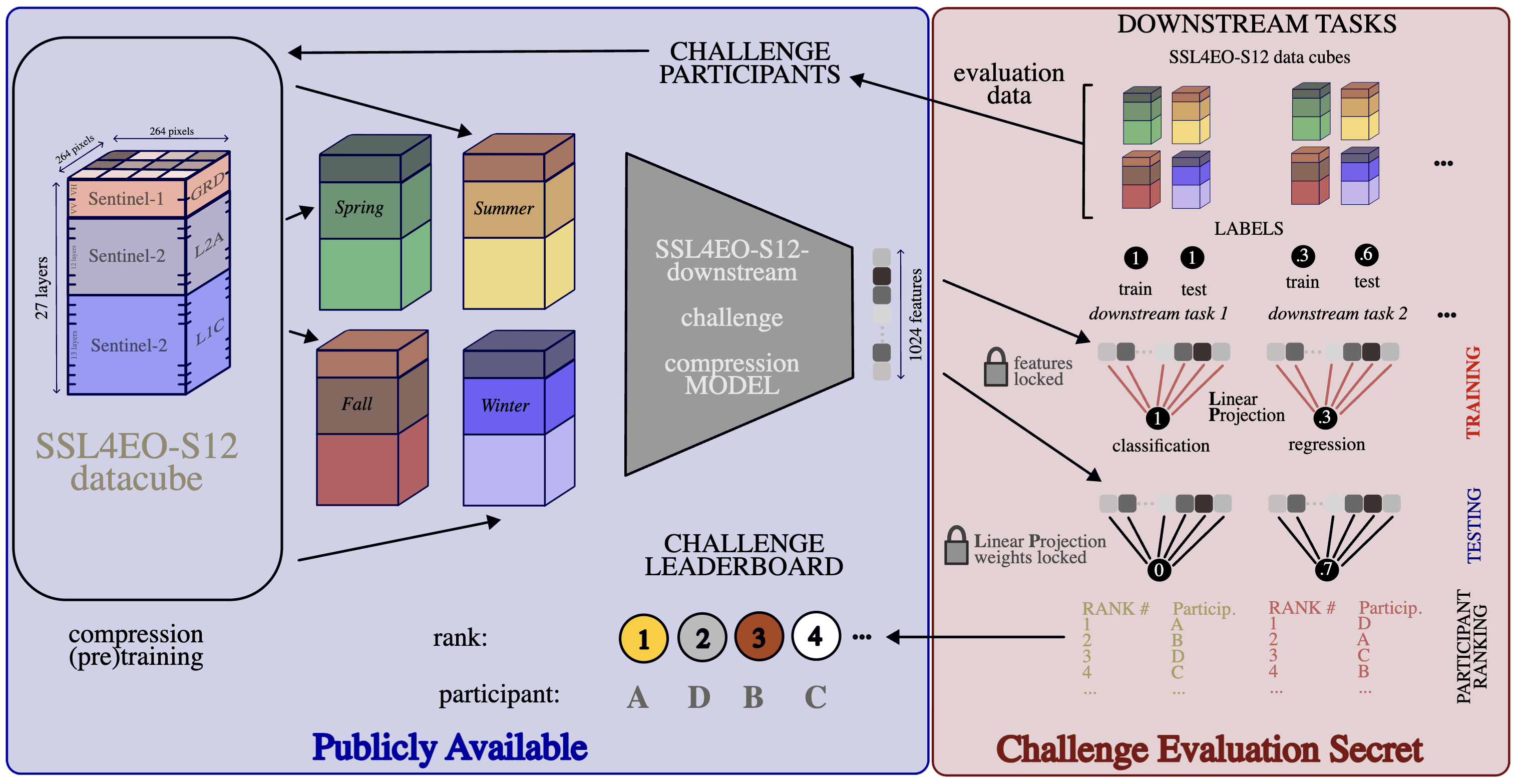
Credit: DLR (CC BY-NC-ND 3.0)
The timeline
The development phase – March 10-31
You will have three weeks in March 2025 to develop your encoder and test the quality of your embeddings by interacting with this challenge portal Eval.AI, submitting embeddings for data available at HuggingFace.
The testing phase – April 3-5
The 1st week of April will allow you three submissions in three days based on a separate dataset made public on HuggingFace two days before. This phase determines the final leaderboard and challenge winner who will present their solution at the workshop in Nashville, TN, USA, or remotely as per availability.
The winners presentation – June 11-12
After the CVPR EARTHVISION has come to a conclusion, the consortium will open-source the benchmark framework to serve the Earth observation compression community to add downstream tasks, and to push the boundaries of neural compression.
Objectives & Impact of the Challenge
Participants will have the opportunity to put cutting-edge compression techniques to the test, as outlined in our recent GRSM review, and stress-test geospatial foundation models that claim to generate generic embeddings for a wide range of downstream applications.
This challenge introduces a novel benchmarking approach, designed specifically for the development and evaluation of Earth Observation foundation models. By assessing their performance across diverse downstream tasks, the initiative aims to provide valuable insights into the effectiveness of AI-powered compression techniques.
The expected impact of the challenge extends beyond technical advancements. It is set to push the boundaries of the state-of-the-art in lossy neural compression, providing a robust framework for assessing the utility of compressed geospatial embeddings. Additionally, it supports the growth of open-source and open-science initiatives, ensuring transparency and accessibility in benchmarking foundation models.
Through this initiative, the geospatial research community can further refine and enhance AI-driven methodologies, ultimately enabling more efficient, scalable, and impactful EO applications.
Link to the challenge and what you need to know: